
Reinforcement learning: the key to autonomous AI
Introduction: Conquering intelligent autonomy
Artificial intelligence (AI) is constantly pushing back the boundaries of what machines can achieve. While classic machine learning, whether supervised or unsupervised, has enabled significant progress, one area is now attracting the attention of researchers, companies, educators and the curious: reinforcement learning. This revolutionary approach places the agent at the heart of the action, confronting it with a dynamic environment where it learns by trial and error, just like a human being exploring the world.
From AI robotics to finance, video games and education, reinforcement learning is becoming a mainstay of modern artificial intelligence, and its potential still seems far from fully exploited.
What is reinforcement learning?
Reinforcement learning (RL) is a branch of machine learning in which an intelligent agent learns to make decisions by interacting with an environment. Unlike supervised learning, where the algorithm is guided by labeled examples, here the agent discovers on its own how to act to maximize a reward.
The principle is simple but powerful: for each action taken, the agent receives feedback from the environment in the form of a reward (positive or negative). His aim is to maximize the accumulation of rewards over the long term, by progressively refining his action strategies, known as policies.

How does reinforcement learning work?
Let's delve into the fine mechanics of this fascinating apprenticeship:
1. The agent
The agent is the entity that acts. It can be a robot, a piece of software, or even a character in a video game. Its purpose: to learn how to achieve an objective in its environment.
2. Environment
The environment is everything that surrounds the agent. It provides information about the current state (called state) and reacts to the agent's actions by generating new states and rewards.
3. The rewards
A reward is a numerical signal indicating the quality of an action in a given state. A positive reward encourages repetition of the action, while a negative reward encourages avoidance of the behavior.
4. The policy
The policy is the strategy adopted by the agent to choose its actions according to the current state. It may be fixed at the outset, but is often optimized over time.
5. Exploration and exploitation
The agent must constantly navigate between two attitudes:
- Exploration: try out new actions to discover their impact.
- Exploitation: use the best known actions to maximize rewards.
Striking the right balance between exploration and exploitation is fundamental to effective learning.
6. Iterations and continuous improvement
Learning takes place in successive iterations: with each interaction, the agent updates its understanding of the world and refines its policy.
A classic algorithm for implementing this learning process is Q-learning. It constructs a Q-table associating an estimated quality value with each pair (state, action), and updates these values according to the feedback obtained.
Key Q-learning formula :

Examples of concrete applications
Reinforcement learning is not limited to theory: it is already shaping our daily lives.
1. AI Robotics
In AI robotics, a robot can learn to walk, grasp objects or avoid obstacles without explicit programming. For example, Learning Robots' AlphAI enables educational robots to learn autonomous behaviors, visible in real time.
2. Video games
Intelligent agents have outperformed human champions in complex games such as Go (DeepMind's AlphaGo) or StarCraft II. They use millions of iterations to discover optimal strategies.
3. Finance
In finance, reinforcement learning algorithms optimize investment portfolios, dynamically adapting buying and selling strategies according to market trends.
4. Autonomous transport
Autonomous vehicles also rely on these algorithms to make optimal driving decisions in complex and unpredictable environments.

Comparison with supervised and unsupervised learning
Supervised learning: the algorithm learns from labeled data (examples/known answers). Example: classify images of cats and dogs.
Unsupervised learning: the algorithm attempts to discover hidden structures without labels (such as groupings).
Reinforcement learning: the algorithm learns to act in an environment by receiving deferred rewards.
Each of these approaches has its own uses. Reinforcement learning is particularly well suited to situations requiring sequential, adaptive decision-making.
The growing importance and future of reinforcement learning
With the rise of artificial intelligence and deep neural networks (deep reinforcement learning), reinforcement learning is gaining in importance. It paves the way for proactive systems capable not only of processing data, but also of acting autonomously and optimally in the real world.
Over the next few years, we expect to see the emergence of even more ambitious applications, in sectors as varied as personalized healthcare, intelligent energy management and education.
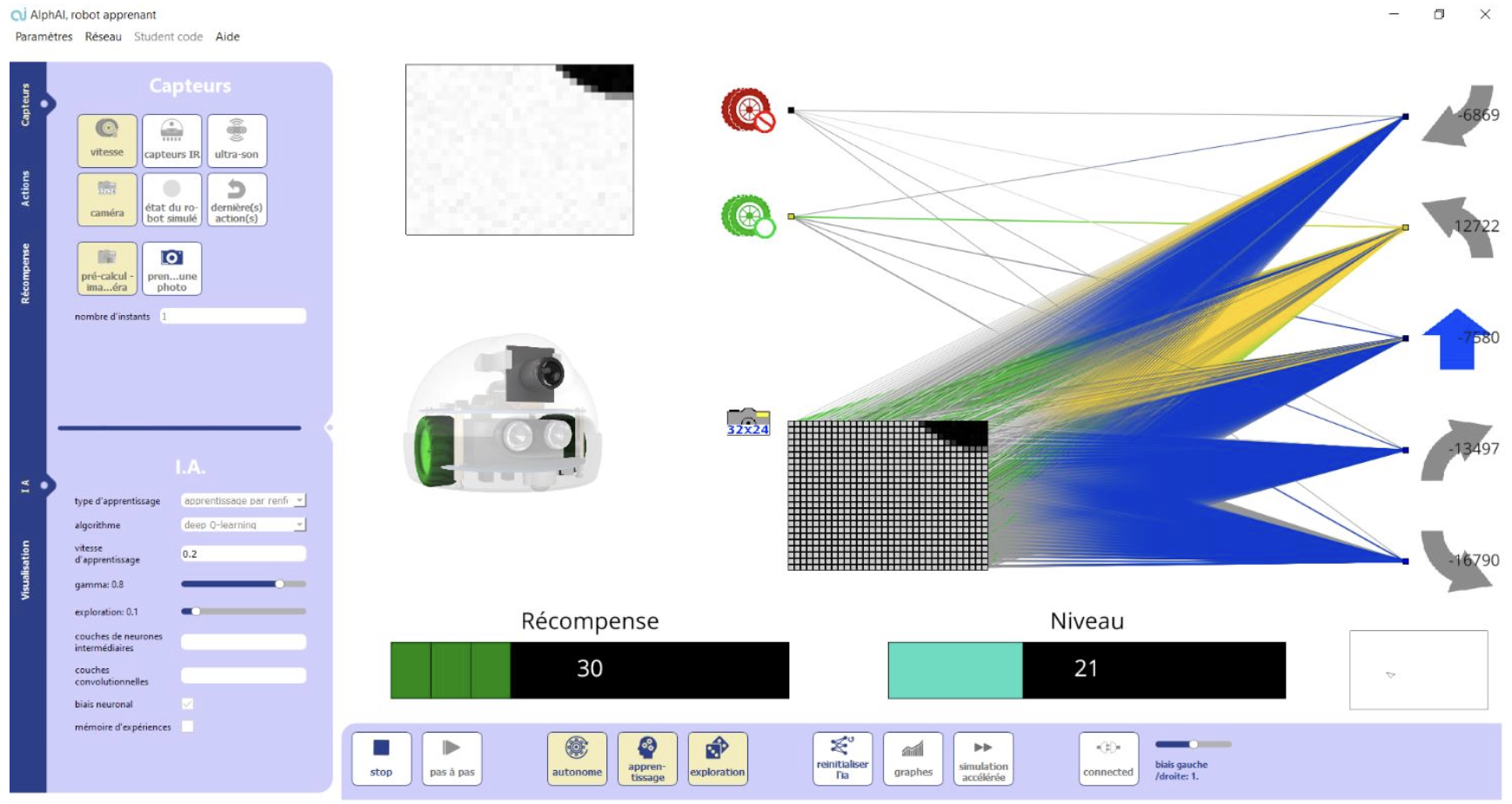
Learning Robots and AlphAI: making reinforcement learning accessible
Learning the subtleties of reinforcement learning can seem complex. That's why Learning Robots offers AlphAI, a unique teaching solution.
With AlphAI, teachers, students and professionals can :
- Visualize reinforcement learning live.
- Manipulate learning algorithm parameters.
- Understand the concepts of exploration/exploitation, reward and politics in a concrete way.
- Program and train real educational robots.
Today, AlphAI is a formidable tool for developing innovative educational AI, combining theory and practice, while demystifying advanced concepts.
Find out how AlphAI makes a key area of artificial intelligence accessible and fun on the page the solution.
Conclusion: Reinforcement learning, the driving force behind autonomous artificial intelligence
Reinforcement learning is profoundly transforming the way machines learn to interact with their environment. From AI robotics to video games, from financial markets to autonomous cars, it is establishing itself as an essential pillar of modern artificial intelligence.
Through concepts such as reward, policy and exploration/exploitation balance, it enables the construction of autonomous agents capable of optimizing their decisions in complex contexts.
Want to put theory into practice?
To experience reinforcement learning in practice and see these fascinating concepts in action, discover our solution AlphAI.
Read also
Dive into AI with our in-depth resources.
.webp)